ASTEM and OVIZIO successfully implemented
a label-free method to monitor human bone marrow MSC morphological profile
Challenge
Mesenchymal stem cells (MSCs) are widely studied as a potential therapy for immune-mediated diseases (osteoarthritis, diabetes, multiple sclerosis, and Alzheimer’s disease). MSCs are known to modulate immune cells such as T cells, B cells, macrophages, microglia, and dendritic cells.
MSCs can be used in an allogeneic, off-the-shelf manner, and have been studied in over 600 clinical trials to date.
However, the manufacture of MSCs with consistent and predictable quality has proven difficult. There is an urgent need to develop imaging systems that are robust and provide real-time monitoring of attributes that are critical to the quality of the stem cells. It is of particular importance that such systems have the ability to not only monitor changes in stem cell phenotype but also their function. Moreover, such a system must also be able to account for donor-to-donor variability.
Solution
Ovizio’s qMod camera captures quantitative phase images from which 30 features related to stem cell phenotype can be extracted (aspect ratio, diameter, elongatedeness, etc). It does so in a label-free manner, easing the burden on quality
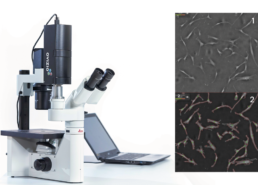
Phase image of MSC culture¹ with mask overlay²
The morphological features can be extracted from phase images and machine learning algorithms can be used to generate phenotypic signatures such as cell count, viability, priming status and immunosuppressive capacity.
Recently, software tools such as t-SNE have been developed to enable easy 2D visualization of complex data, facilitating exploratory research and potential identification of unknown populations.
Experimental Set-Up
We succeeded in establishing the optimal MSC seeding density and priming conditions to capture morphological changes in MSCs isolated from three donors.
We developed a preliminary algorithm to identify multiple MSC morphological features (cell aspect ratio, cell area, elongatedness, optical height) to predict the effects of IFN-γ and TNF-α priming. These observations show a
strong dependence on cell density, irrespective of donor.
Results
Applying the t-SNE algorithm on the data revealed subpopulation differences for all donors. When primed, there is an increase in MSC subpopulation 1 that corresponds to larger, more elongated, and flatter cells with a higher aspect ratio.
t-SNE algorithm testing on unprimed
and primed MSCs
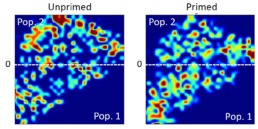
The distribution of the four cell features in Donor 1 is illustrated above. Even though each donor has the same qualitative differences between Pop. 1 and 2, the feature distribution is donor-dependent.
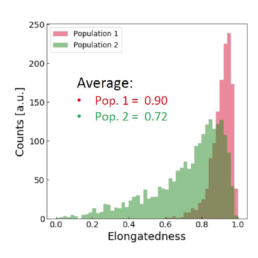
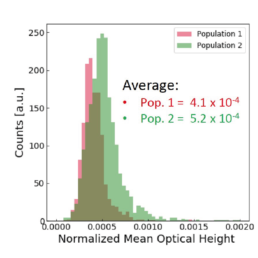
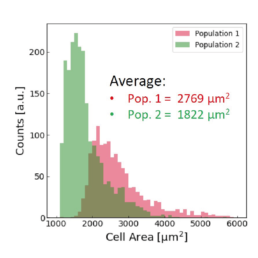
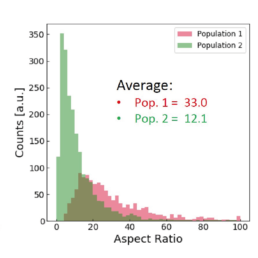
Using all available data, we built a supervised machine learning model (random forest classifier with shallow trees) using the computed cell features as input for the model. The preliminary supervised machine learning model concluded that the effect of priming on MSCs is not dependent on the donor. Rather our study showed:
- an increase in the cell aspect ratio (length/width)
- an increase in the cell area
- an increase in the elongatedness
- a decrease in the optical height
Supervised machine learning model testing on unprimed and primed MSCs
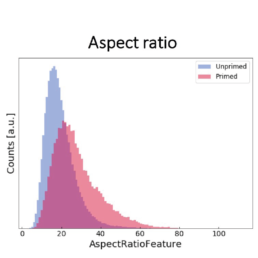
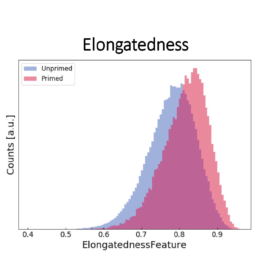
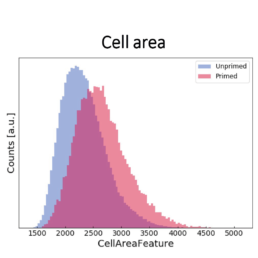
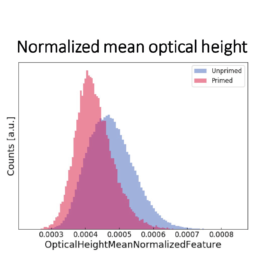
The image-based prediction model showed the distribution of the four cell features in unprimed (blue) and primed MSC populations (pink).
Conclusion
We developed a machine learning algorithm to predict the effect of priming on changes in MSC morphology. We show that primed MSCs are larger, more elongated, and have a flatter and higher aspect ratio.
These results will be further validated in a comprehensive study using MSCs isolated from 12 additional donors. This will include a correlation between MSC function and morphological changes in response to various priming events. Ultimately, this study seeks to position the qMod imaging system for monitoring the quality of MSCs in the manufacturing workflow.
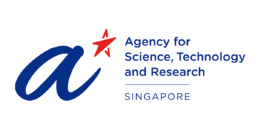
Contact: Pascale Charbonnel, pascale.charbonnel@ovizio.com
Catherine Chong, chongyf@imcb.a-star.edu.sg
Steve Oh, Simon Cool, Ying Zhang, Yin Lu, Tongming Liu from ASTARSG
Damien Cabosart, Pascale Charbonnel, Emilie Viey from Ovizio